The Need
Agriculture is fundamental to the livelihoods of billions of people worldwide. AI-enabled tools have great promise to increase production and resilience and contribute to broader sustainable development goals, but often crucial training data is not available in the public square.
In the agricultural AI for social good domain, recent advances in the analysis of remote sensing data have enabled improved accuracy of a variety of AI tasks. Both startups and large development programs have built on this data availability to provide personalized information and predictive services for farmers.
However, there is a lack of accurate ground truth labeled datasets in a variety of geographies in the Global South. Techniques and datasets to address unique challenges in mapping intercropping and smallholder farms are out of reach. This hinders progress in building beneficial ML applications that are scalable and robust for populations worldwide.
Alongside an explosion of earth observation providers and services, nascent standards, such as the Spatio Temporal Asset Catalog (STAC) and a preliminary version of best practices for ground reference data collection and cataloguing are creating greater coherency for machine learning-ready training data across the AI for agriculture landscape.
Datasets for Machine Learning Diagnostics in Livestock
Lacuna Funding
To complement and expand these efforts, Lacuna Fund supports the creation, expansion and maintenance of training and evaluation data in the agriculture space. Our Technical Advisory Panel – who is responsible for identifying data gaps, developing the call for Expressions of Interest, reviewing and identifying promising applications to be invited to respond to the RFP, and reviewing and selecting candidates for funding – has identified needs for labeled datasets in the following application areas. However, Lacuna Fund’s call for EOIs and RFPs are intentionally open, to encourage new and innovative ideas that we may not have identified.
Soil, Water and Crop Management
- Soil properties prediction;
- Water and irrigation management;
- Yield estimation;
- Crop type classification;
- Crop protection;
- Weed management;
- Prediction and early warning on the anticipated conflict between pastoralists and crop farmers.
Livestock Management
- Breeding and livestock breeds dataset;
- Livestock management;
- Feed optimization and forage availability prediction;
- Livestock diseases and management;
- Prediction and early warning on the anticipated conflict between pastoralists and crop farmers;
- Management of pastoral migratory patterns among others.
Cross-cutting categories
- Inputs supply and demand management;
- Produce supply chain management;
- Produce aggregation and demand management;
- Input and produce marketing and distribution management;
- Produce processing;
- Extension service provision.
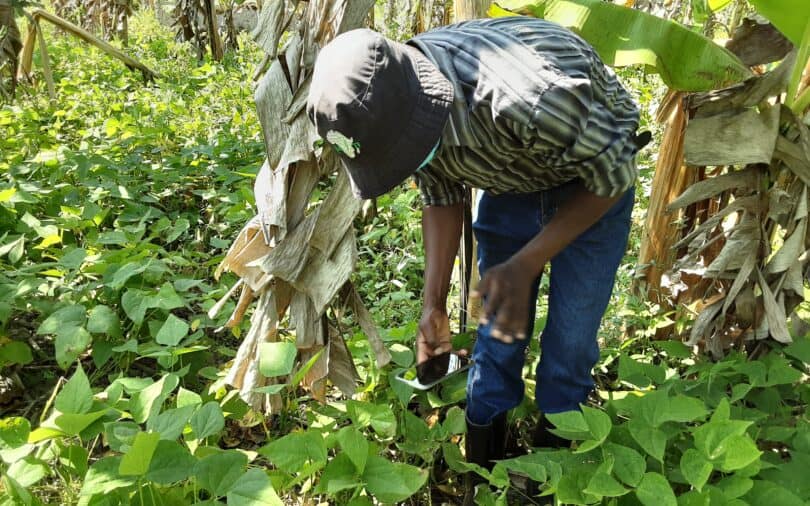