The Need
The value of machine learning (ML) in healthcare is its ability to process huge datasets beyond the scope of human capability, and then reliably convert analysis of that data into insights that aid health professionals in planning and providing care, ultimately leading to better outcomes, lower costs of care, and increased patient satisfaction. [1]
In many settings, particularly those that are low- and middle- income, ML is not utilized for healthcare, often due to the unavailability of datasets and a lack of infrastructure to support the implementation of ML applications. In addition, healthcare datasets often lack broad representation across demographic and socioeconomic groups. Therefore, where ML is utilized, the datasets that inform the algorithms supporting diagnosis and treatment risk being biased. Where data is representative, the data may also not be accurate, resulting in the incorrect understanding of differences across groups. Current data may also miss important socioeconomic, environmental, and other data that can determine health outcomes. Improvement of these datasets may improve ML models and ultimately, health outcomes across populations, from prevention, to diagnosis and treatment. [2]
Sexual, Reproductive and Maternal Health and Rights (SRMHR) are also crucial for the health and survival of people across all genders, as well as to social and economic development. Well-designed SRMHR interventions have proven to be extremely cost-effective. However, the SRMHR of many people, particularly in LMICs, are far from realized.
Lacuna Funding
Lacuna Fund supports dataset creation, aggregation, and maintenance for the training and evaluation of machine learning models to improve health datasets and outcomes in two tracks:
- Addressing inequities in healthcare outcomes in the United States and in low- and middle-income contexts globally
- Improving Sexual, Reproductive, and Maternal Health and Rights
We seek datasets by local communities designed to address locally-identified needs, so the following are illustrative examples only. The TAP and Lacuna Fund welcome proposals outside these topics:
- Question and answer datasets with high quality, evidence-based medical information validated by healthcare professionals, used to train chatbots, conversational agents and other applications focused on information provision.
- Image datasets to train artificial intelligence and support screening and diagnosis.
- Large population datasets (including longitudinal) such as maternal health datasets, civil registration and vital statistics, and longitudinal HIV datasets among others.
- Datasets representing the experience in the treatment process.
- In all datasets: gender-responsiveness and inclusion of key vulnerable groups
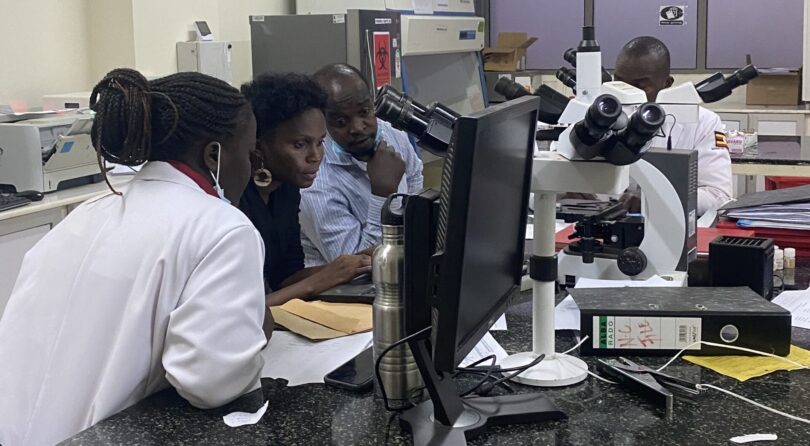
Explore the Data
[1] Corbett, Ed. “Real-World Benefits of Machine Learning in Healthcare.” Health Catalyst, 19 Nov. 2020, www.healthcatalyst.com/clinical-applications-of-machine-learning-in-healthcare.
[2] Wawira Gichoya J, McCoy LG, Celi LA, et al. “Equity in essence: a call for operationalising fairness in machine learning for healthcare”. BMJ Health & Care Informatics 2021;28:e100289. doi:10.1136/bmjhci-2020-100289