The Need
The ability to communicate and be understood in one’s own language is a prerequisite to digital and societal inclusion. Natural language processing (NLP) techniques have enabled critical applications to achieve this—to improve education, financial inclusion, healthcare, agriculture, communication, and disaster response, among many other areas.
However, a gap in openly accessible datasets outside of English and other Indo-European languages has prevented breakthroughs based on NLP technologies. Labeled data and speech corpora remain a key element of this gap, as well as the availability of corpora that can be used in transfer learning or semi-supervised approaches.
NaijaVoices: Curation of Speech Datasets in Igbo, Hausa, and Yoruba
Lacuna Funding
Lacuna Fund’s efforts in NLP build on a recent groundswell of momentum to create better and more open NLP tools in underserved languages from ML community members, including recent academic workshops, volunteer collaborations, innovative academic programs, and other efforts.
To complement and support these efforts, Lacuna Fund supports open training and evaluation datasets for NLP in underserved languages. Our Technical Advisory Panel (TAP), who is responsible for identifying data gaps, developing the RFP, and reviewing and selecting proposals, has identified needs for labeled datasets in the following areas. However, Lacuna Fund RFPs are intentionally open, to encourage new and innovative ideas that we may not have identified.
The TAP sees a need for datasets that enable better execution of core NLP tasks in African languages, including but not limited to the following:
- Speech corpora, particularly enabling automated voice recognition that allows illiterate or otherwise underprivileged groups of persons to access information and/or services;
- Labeled and unlabeled text corpora for use as training data;
- Parallel corpora for machine translation;
- Corpora to support fundamental NLP tasks, such as named entity recognition (NER), part of speech tagging, embeddings, etc.;
- Datasets for key downstream NLP tasks, such as question answering and conversational AI, sentiment analysis datasets, or technology for language education;
- Datasets to improve the performance of NLP tasks on code-switched text or speech.
More broadly, there is also a need for:
- Augmentation of existing datasets in all areas to decrease bias (such as gender bias or other types of bias or discrimination) or increase the usability of NLP technology in low- and middle-income contexts;
- More benchmark data for NLP tasks in underserved languages or to inform multilingual models;
- Innovative datasets, such as video or audio captioning or other image-text interactions;
- Domain-specific creation or augmentation of text and speech datasets, such as digit datasets, place names, or specific word pairs or sentences, that enable applications with significant social impact.
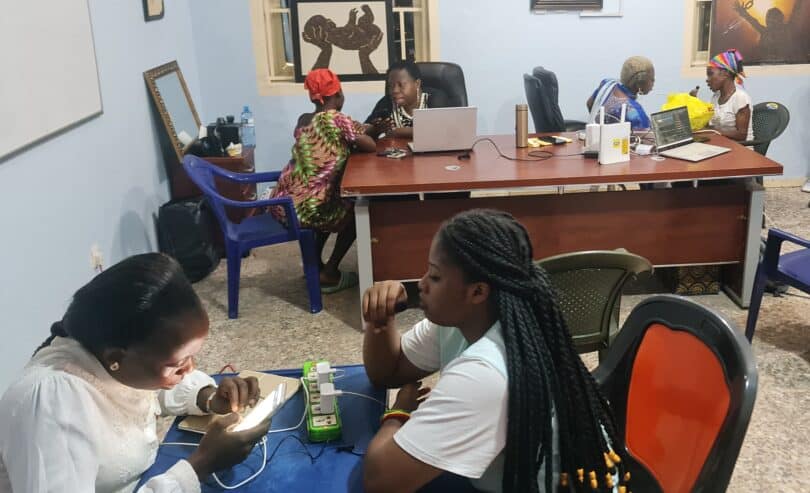