Four New Machine Learning Datasets in Agriculture, Health, and Language Domains
28 March 2024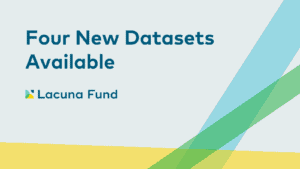
Four New Machine Learning Datasets in Agriculture, Health, and Language Domains
Today, we are excited to announce four recently published datasets for training artificial intelligence in the domains of Agriculture, Natural Language Processing (NLP), and Health. These datasets harness the power of AI to address urgent social and economic problems across several African countries.
These four featured datasets include:
- A robust dataset mapping communal resources in pastoral regions of Northern Tanzania: this team has collected extensive data on key livestock resources and mapped out livestock migration patterns. This dataset will provide invaluable insights, illuminating the current conditions of pastoralist communities and their adaptation strategies. These insights will be instrumental in formulating targeted interventions to effectively support these communities. Moreover, it will streamline the development of community-driven land use plans, thereby reducing conflicts between herders and farmers that arise from livestock migration.
- Makerere University NLP Datasets: this project team has created text and speech datasets for low-resourced East African Languages (Uganda, Tanzania, Kenya). It has also increased the available monolingual or parallel corpora for Swahili, Luganda, Runyankore-Rukiga, Luo/Acholi, and Lumasaaba for building NLP applications.
- A machine-learning dataset for rabies diagnosis and outbreak prediction: this diagnostic dataset can help create machine-learning binary classification algorithms to predict if a human or animal has rabies and can provide real-time and remote diagnoses in low-resource settings. The team used data from an existing rabies surveillance system (Integrated Bite Case Management) and have published a machine-learning-ready dataset to apply AI solutions to rabies control in Africa.
- Enhanced Agriculture Datasets for Remote Crop Monitoring to Provide Access to Essential Social and Financial Services to Smallholder Farmers in Zimbabwe: this team has generated and enhanced labeled, remote-sensing, and field datasets in Zimbabwe during growing and harvest seasons. They used machine-learning models to evaluate risks and gain insights into weather, agroecological conditions, and farming practices’ effect on yield and overall productivity. Their primary aim is to provide African farmers access to fairly priced insurance and credit services and improve their resilience to an increasingly volatile climate.
We extend our deep gratitude to our Funders who made the creation of these datasets possible:
- Canada’s International Development Research Centre (IDRC): 2020 Agriculture, 2020 Language, 2021 Agriculture
- Google.org: 2020 Agriculture, 2020 Language, 2021 Agriculture
- The Rockefeller Foundation: 2020 Agriculture, 2020 Language, 2021 Agriculture
- Wellcome Trust: 2021 Equity & Health
See below to access these datasets and find out more about what exactly is contained within each of them!
A Decision-Supporting Tool for Developing Community-led Land Use Plans
Contact: Gladness Mwanga|gladnessg@nm-aist.ac.tz and Divine Ekwem |divine.ekwem@glasgow.ac.uk
This dataset focuses on locations with predominantly pastoral communities in northern Tanzania to identify fine and broad-scale movements of livestock and land use patterns and to understand how these relate to communal conflicts. It is a high-quality, accurate and labeled (image, location, and time stamps) dataset containing detailed information on ~ 2000 communal resources (e.g., rangelands, water points, and dips) and their use patterns for over 220 villages across four large districts in northern Tanzania, representative of pastoral systems of livestock production in East Africa. The dataset can be used to describe forage and livestock resource management in managed ecosystems such as community rangelands; identify major migration routes among pastoralist herds and the location and type of infrastructure required to support livestock production; anticipate the location of conflicts with crop farmers and determine the best locations to establish forage banks and support infrastructure along livestock migratory routes.
Authors and Affiliations: Dr. Divine Ekwem (University of Glasgow); Gladness Mwanga (Nelson Mandela African Institution of Science and Technology), Professor Gabriel Shirima (Nelson Mandela African Institution of Science and Technology), Professor Mizech Chagunda (University of Hohenheim)
Dataset: access here.
Makerere University NLP Datasets
Contact: Andrew Katumba | andrew.katumba@mak.ac.ug
Makerere University has created text and speech datasets for low-resourced East African Languages in Uganda, Tanzania, and Kenya. This dataset contains 10,000 parallel sentiment-tagged sentences, 100,000 Kiswahili sentences, 100,000 Luganda sentences, 40,037 Acoli sentences, and 39,999 Lumasaaba sentences. On Common Voice, the text dataset comprises 100,000 Luganda sentences and 100,000 Swahili sentences. The text datasets can be used for building machine translation, next-word predictor/auto-completion, topic modeling and classification, sentiment analysis, and language models. The Luganda and Swahili voice datasets can empower entrepreneurs to innovate around existing gaps in their communities to build systems for visually impaired or physically handicapped people, native language tutors, medical transcription tools, and more. Application developers interested in translation engines, text editors, and text and grammar spelling systems in the East African community will benefit from the datasets.
Datasets:
- Text data: access here.
- Luganda voice data: access here.
- Swahili voice data: access here.
Authors and Affiliations:
- Makerere University: Katumba Andrew, Nakatumba-Nabende Joyce, Babirye Claire, Mukiibi Jonathan, Tusubira Jeremy, Bateesa Tobias, Wairagala Eric Peter, Fridah Katushemererwe, Mutebi Chodrine, Nabende Peter, Sentanda Medadi, Ssenkungu Ivan
- Wanzare Lilian (Maseno University)
- Davis David (TYD Innovation Incubator)
- Okidi George
- Ayugi Carolyne
- Muzaki Naomi
Machine Learning Dataset for Rabies Diagnosis and Outbreak Prediction
Contact: Asa Emmanuel | asakalonga@gmail.com and Kennedy Lushasi | klushasi@ihi.or.tz
This dataset will help in the real-time and remote diagnosis of rabies disease for humans and animals in low-resource settings. A time series approach can be applied to the outbreak dataset to predict the number of rabies cases likely to occur within an area after a given time interval. This approach can help with resource mobilization, too, such as identifying the number of vaccines required in a specific area at a given time. The number of observations from the two datasets is 12,684. There are three datasets for rabies diagnosis for animals and humans, with 7,081 and 4,585 observations, respectively. In the outbreak prediction dataset, 1,018 observations were accounted for.
Authors and Affiliations: Asa Emmanuel, Rebecca Chaula, Deogratias Mzurikwao, Joel Changalucha, Kennedy Lushasi
Dataset: access here.
Enhanced Agriculture Datasets for Remote Crop Monitoring to Provide Access to Essential Social and Financial Services to Smallholder Farmers in Zimbabwe
Contact: Seth Odhiambo | sodhiambo@pula.io
The project created labeled yield estimates from 3000 farmers, and was used to train prediction models for yield prediction across the country, consequently using the dataset to generate high resolution crop mask layers for the different value chains. The yield prediction models were enhanced by other biophysical datasets ranging from soil properties and climate related indicators. The datasets proved a concept of scalable machine learning models training, which may be able to respond more appropriately and cost-effectively to agricultural stressors, thereby ensuring a positive impact on agricultural practices (e.g., good agricultural practices), yields (e.g., harvest quality and quantity), and farmer access to financing (e.g., crop insurance).
Authors and Affiliations: Pula Advisors
Dataset: access here.
Background:
Why do we need more open datasets in the domain of natural language processing (NLP)?
Timely and accurate access to information – spoken or written – in one’s own language is key to being able to fully participate in the digital world. Translations, the ability to understand and synthesize speech, and many other AI-enabled applications in the field of natural language processing (NLP) require training and evaluation data that does not exist for many low-resourced languages, some spoken by millions of people around the world. Therefore, Lacuna Fund supports the creation of open training and evaluation datasets for NLP in underserved languages. Learn more here.
Why do we need more open datasets in the domain of Agriculture?
Lacuna Fund agriculture datasets unlock the power of machine learning to alleviate food security challenges, spur economic opportunities, and give researchers, farmers, communities, and policymakers access to superior agricultural datasets. Learn more here.
Why do we need more open datasets in the domain of Health?
Lacuna Fund aims to close the gap in health disparities by fostering interdisciplinary collaborations that create, expand, or aggregate labeled training and evaluation datasets. Ultimately, this information aims to help providers and patients make decisions that lead to more equitable healthcare outcomes. Learn more here.