Announcing Supported Projects in Agriculture
15 June 2022Today, we are proud to share five newly supported projects in the agriculture domain. From rangelands in Namibia to smallholder farms in Zimbabwe, the teams selected for funding are driving progress in the agriculture sector on food security, economic development, and more across Sub-Saharan Africa.
We thank our partners at ECI-Africa who facilitated the Agriculture Technical Advisory Panel. In addition, we extend our deep gratitude to our 2021 Agriculture Technical Advisory Panel and partner reviewers for their work distilling a rich application pool and selecting a diverse portfolio of projects for funding:
- Drew Wheadon, Tetra Tech ARD
- Hamed Alemohammad, Radiant Earth Foundation
- Louise Donnison, University of Edinburgh
- Meareg Hailemariam, Dakar American University of Science and Technology
- Ruthie Musker, Centre for Agriculture and Bioscience International (CABI)
- Zara Khan, Delhi Technological University
We also owe great thanks to our funders who supported this call: The Rockefeller Foundation, Google.org, Canada’s International Development Research Centre (IDRC), and the German development agency GIZ on behalf of the Federal Ministry for Economic Cooperation and Development (BMZ).
In our 2021 application cycle, the agriculture domain saw a plethora of interest from over 75 applications from, or in partnership with, organizations across Africa. Almost two years after Lacuna Fund’s launch, it is heartening to see such strong engagement. Read on to learn more about the most recent round of projects selected for funding!
Drone-Based Agricultural Dataset for Crop Yield Estimation
This project by KaraAgro AI in collaboration with Makerere AI Lab will create a dataset suitable for crop type classification and detection, fruit maturity stage classification and detection, and yield estimation. Using drones, the team will collect a total of 12,000 aerial images of trees and fruits from Ghana and Uganda—including 6,000 aerial images of cashew trees and fruits from Ghana and Uganda, 3,000 aerial images of cocoa trees and fruits from Ghana, and 3,000 aerial images of coffee trees and fruits from Uganda. The team will annotate the trees with appropriate crop type labels and visible fruits with appropriate labels representing the stage of development.
This project takes us closer to transforming African agriculture into agribusiness… the dataset will allow for the development of yield estimation solutions that will provide farmers with an opportunity to make good business decisions and appropriately plan ahead towards their equipment, fuel and labor needs, ensure they have enough storage available, cash-flow budgeting, and make early marketing decisions. It’d also allow for timely harvest, which provides farmers the opportunity to ensure healthy and fresh produce, and therefore better sales and income.”
– Darlington Akogo, Founder, CEO, KaraAgro AI and the project’s Principal Investigator
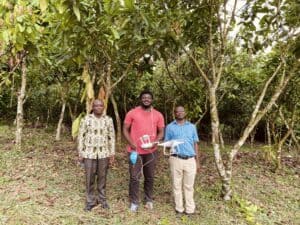
Enhanced Agriculture Datasets for Remote Crop Monitoring to Provide Access to Essential Services to Smallholder Farmers in Zimbabwe
This project will build yield maps localized to certain regions in Zimbabwe, focusing on maize, soybeans, and wheat, in order to increase access to agriculture insurance, credit, and digital extension services and improve food security monitoring and responses. Utilizing Android smartphones/tablets and a custom application to ensure quick, high quality data processing and analysis, the team will collect data points from 3,000 smallholder farmer households. The project will also create a data visualization dashboard that presents actionable information and maps to stakeholders to support decision-making about product development and marketing, disaster mitigation, public financing, and policies.
We are thrilled to be implementing a project that will enable accurate farm-level analytics for smallholder farmers, helping to solve some of their main concerns including access to affordable financial services. Their income and productivity will increase over time as a result of better targeted services and access to data-driven agronomy services.”
– Mwenda Mugendi, Applied Research Manager, Pula Advisors
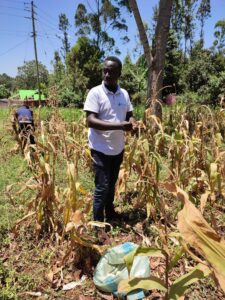
Mobile ELISA and Syndromic Datasets to Enable Rapid Machine Learning Automation for Pen-Side Disease Diagnostics in Livestock
This project will create livestock datasets for disease identification, geospatial epidemiological modelling, and rapid point-of-care diagnostics in low-resourced rural remote areas. The datasets will unlock research for the livestock sector and reduce the cost of access to accurate infield diagnosis with model deployment to mobile smartphones. This will create opportunities for mass livestock screening even in the most remote herding regions that are severely limited by costly cold chain logistics to highly capable laboratories. In addition, the datasets of geotagged and expert-labelled symptomatic images will form the basis of real-time symptomatic surveillance mapping, enabling national-level livestock agencies to monitor incidence and severity of symptoms/disease at scale and inform evidence-based intervention planning.
With support from Lacuna Fund, the joint efforts of Makerere AI Lab, Makerere Molecular Lab, and Veterinarians Without Borders will pave the way for open datasets for machine learning, an important contribution to innovation for resilience of the livestock sector in Africa and emerging economies at large.”
![]()
A New Rangeland and Pasture Management Open Dataset for Namibia
Namibia’s rangelands and ecosystems are eroding and degrading more than ever, threatening local biodiversity and food security. Aiming to counter these issues and empower communities to better manage, plan and mitigate rangeland health, this project team will create a dataset by monitoring different regions in the country and combining three types of georeferenced data collection. The collected data will help estimate the carrying capacity of a specific area in a selected period, forecast pasture biomass expected to be available following the rainy season, estimate the level of bush encroachment and its distribution in selected areas, and predict grazing vegetation biomass during the growing season.
We are driven by the positive impact that this first dataset will bring to Namibia’s rangeland and pasture management. We look forward to collaborating with a network of local farmers, workers, and supervisors interested in the development of the machine learning algorithms to extract the much-needed information in this poorly studied semi-desertique region where locals depend on rangelands for food security and livelihoods.”
– Andrea Capobianco Dondona and the Farm4Trade team
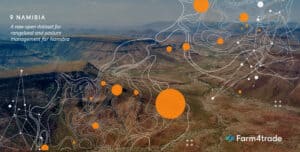
A Region-Wide, Multi-Year Set of Crop Field Boundary Labels for Sub-Saharan Africa
Comprehensive maps of crop field boundaries do not exist for most countries in Africa, resulting in a lack of labeled images needed to train and validate mapping models—despite the increasing availability of high-resolution satellite and deep learning models. This project will develop a comprehensive, high-quality label set that will be immediately useful for training generalizable, region-wide field boundary mapping models, and for refining and validating models for specific regions and years. The team will label the fields in images collected across Sub-Saharan Africa during the primary growing seasons over 6 years.
There are no publicly available field boundary labels that cover Sub-Saharan Africa’s broad range of agricultural systems… [our] completed label set can be put to immediate use in building improved cropland mapping models. Its comprehensiveness will enable generalizable models, trained over the entire region and time domain, to be developed, and also allow models to be locally refined and validated within specific sub-regions and years.”