Announcing Awards for Health Datasets
19 May 2022Today, we are proud to share Lacuna Fund’s first round of awards to create datasets in the health domain. The teams selected for funding are unlocking the power of machine learning across the globe, from the rural provinces of Nepal to the neighborhoods of Chicago, USA. This funding and the project teams’ work will create, augment, and aggregate open datasets that are representative of affected populations and are therefore less biased and more likely to lead to equitable health outcomes worldwide.
We extend our deep gratitude to our 2021 Health Technical Advisory Panel and partner reviewers, who were instrumental in selecting projects poised for impact across communities, sectors, and the world:
- Dr. Alistair Johnson, Hospital for Sick Children
- Chinasa T. Okolo, Cornell University
- Dr. Clement Adebamowo, University of Maryland School of Medicine
- Dr. Curtis P. Langlotz, Stanford University
- Dr. Ivor Braden Horn, Google
- Dr. Mahlet (Milly) Zimeta, Open Data Institute
- Dr. Sanmi (Oluwasanmi) Koyejo, University of Illinois at Urbana-Champaign
- Sekou L. Remy, IBM Research – Africa
We also owe great thanks to our funders who supported this call for proposals—including The Rockefeller Foundation, Google.org, Wellcome Trust, Gordon and Betty Moore Foundation, Patrick J McGovern Foundation, and Robert Wood Johnson Foundation.
This call aimed to address inequities in healthcare outcomes in the United States and in Low- and Middle-Income Countries (LMICs) globally. From a pool of over 60 applications, the teams selected for funding will support a range of needs in the healthcare sector, driving progress on medical diagnoses, childhood malnutrition, chronic pain management, and more.
We are ever inspired by the community-led movements towards locally developed and owned datasets that unlock AI to deliver tangible solutions around the globe. And without further ado, read on to learn more about the most recent round of projects selected for funding!
Supported Project Teams in the Health Domain
AI-Assisted Smartphone Microscopy for Automatic Detection of Diarrhea-Causing Parasites
This project will create a dataset of smartphone-based low-cost microscopy system images to enable real-time automatic detection of diarrhea-causing protozoa, even in places lacking experts or traditional expensive microscopes. Diarrhea is the second leading cause of death for children under five years old globally, with the majority of deaths occurring in LMICs. Using traditional microscope and smartphone-based microscopes in vegetable, water, and human stool samples from provinces of Nepal, the team will create a new annotated dataset of Cryptosporidium and Giardia (oo)cysts. To provide benchmark results, they will also implement and evaluate state-of-the-art deep learning methods for the automatic detection of (oo)cysts of Giardia and Cryptosporidium on an independent test set that will be released as part of the dataset.
We are excited to start this multidisciplinary project that involves researchers and professionals from so many different fields, including AI researchers, microscopy and optics experts, chemistry and low-cost device experts, clinicians, pathologists, public health experts etc.”
– Bishesh Khanal, Principle Investigator, NAAMII
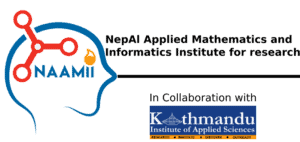
Datasets for AI-Based Diagnosis of Malaria
This project aims to generate quality, accessible, and open-labeled datasets of thick and thin blood smear images from Uganda and Ghana that will contribute to improved malaria microscopy diagnosis. The team will develop standardized data collection protocols for image data acquisition using smartphone cameras mounted on the eyepiece of the microscope. Through their partnership with local health centers, this project will build opportunities for the machine learning community to develop AI-based models for malaria parasite detection, species identification, and malaria parasitemia determination.
Makerere AI Lab, Uganda in collaboration with MinoHealth AI Lab, Ghana, will provide accessible datasets of microscopy thick and thin blood smear images for improved malaria microscopy. Relying on free and open-source datasets will unlock opportunities for the development of reliable and more accurate machine learning models for malaria diagnosis beyond its current standing, thus achieving SDG goal 3: ‘Ensuring healthy lives and promoting well-being for all at all ages.’ We are excited about this project and grateful to the Meridian Institute for giving us this unique opportunity through Lacuna Fund.”
– Rose Nakasi, Makerere AI Lab, Datasets for AI Based detection of Malaria Project Team
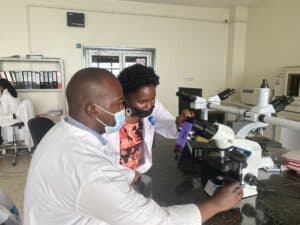
Expanding BraTS Data to Capture African Populations (Africa-BraTS)
This project will create an annotated database of MRI images from sub-Saharan Africa for diagnosis of glioma, a rare, fast growing and highly fatal brain tumor. Over the past 10 years, the Brain Tumor Segmentation (BraTS) Challenge has provided open and accessible high quality labeled MRI images for development of ML tumor segmentation models and benchmarking of model performance. However, it is unclear how these methods translate in low-resourced regions, particularly in sub-Saharan Africa, where glioma mortality rates are the highest and the sustained use of less advanced MRI technology coupled with limited skilled personnel make glioma diagnosis challenging. The Africa-BraTS database pooled from existing patient data will provide a novel way of benchmarking performance of ML models in providing solutions to real world imaging challenges from low-resourced settings. In the end, this will influence how well brain tumors are delineated, measured, and characterized and long-term patient survival.
Our primary aim is to solve challenges that reinforce disparities in application of ML in image-based diagnosis in lower-resourced settings, focusing on challenges unique to Sub Saharan Africa.”
– Udunna Anazodo, Montreal Neurological Institute, Chair, Consortium for Advancement of MRI Education and Research in Africa (CAMERA)
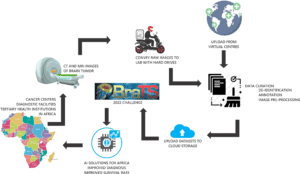
Machine Learning from Real Patient Outcomes to Reduce Racial Disparities in Chronic Pain
This project will build a new, large imaging dataset linked to rich, real patient outcomes, including their pain scores—helping to address underlying pain for underserved populations. By training an algorithm to look at knee X-rays and predict pain that patients report feeling, rather than a doctor’s score of the X-ray, the project team has unlocked medical insights that have been able to better detect causes of knee pain in Black patients. However, the challenge to scaling this approach is that existing datasets are insufficient, as they routinely contain just the knee X-ray and the doctor’s judgment of it. Using this new method, the team will use the unique advantages of AI to sidestep biases inherent in old ways of reading X-rays, and instead produce new knowledge complementary to that of the doctor.
Chest X-Ray Imaging Dataset for Multiple Cardiorespiratory Diseases
Cardiorespiratory diseases are recognized as serious, worldwide public health concerns that have remained among the leading causes of death globally. However, there are not many publicly available datasets from Africa—making it difficult to determine whether tools and techniques developed in other geographies are as effective in this context. This project team will create an open, labeled chest X-ray dataset for multiple cardiorespiratory diseases in Ethiopia, stimulating researchers and practitioners in Africa, adapting current methods to the African context, and building assistive technologies that empower radiologists.
We envision this dataset to have an impact primarily in research and applications of the medical imaging domain. It will also be essential for researchers in natural language processing and entrepreneurs in medical imaging.”
Reducing Childhood Malnutrition in Chile through an Integrated, Multidimensional Database
This project will create an integrated database for child nutritional status, socio-economic and demographic characterization, student academic performance, and health care use and costs related to childhood undernutrition, overweight, and obesity in Chile. Childhood malnutrition is a pathologic process with multidimensional causes that increases the risk of being diagnosed with chronic health conditions, raises healthcare expenditures, reduces productivity, and leads to premature mortality. This team will support the creation of both the dataset and the base infrastructure to make it safely accessible to researchers and policymakers while protecting privacy.
Early life malnutrition can cause permanent deficits in growth and development, and result in several health complications over the life span. Chile has the fourth highest rate of childhood obesity in the Americas, and its prevalence is higher in vulnerable communities. In addition, COVID-19 has disrupted food access and impacted food insecurity. With the support of Lacuna Fund, an interdisciplinary team of data scientists and researchers will integrate different databases that will enable applied research to help policymakers focus on the population at particular risk, build predictive systems that contribute to prevent those risks, and develop interventions that efficiently elicit behavior change.”
– Nieves Valdés, Principal Investigator, Reducing Childhood Nutrition in Chile Project Team
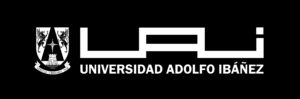
Smartphone Artificial Intelligence Platform for Paper Record Digitization
This project focuses on developing and utilizing a computer vision, deep learning AI platform to convert perioperative paper health records into an electronic database. Paper-based charting precludes adequate characterization, annotation, analysis, and preservation of data records—thereby diminishing their utility in reducing perioperative morbidity and mortality through structured quality improvement interventions and outcomes-based research. Through this project, photographs of intraoperative anesthesia paper health records will be taken by the healthcare provider using a low-cost smartphone. The image will be segmentally deconstructed and digitized using computer vision models creating a labeled dataset. Perioperative digital datasets will then enable healthcare practitioners to further develop models to identify high risk predictors for postoperative complications that are relevant and specific to LMICs.
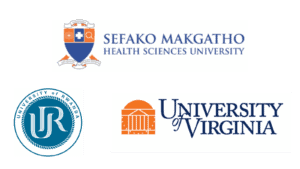
Towards a Machine Learning-Ready Tuberculosis Chest X-Ray Database for Africa
This project aims to create an open, labeled dataset for chest X-ray images and clinical information collected from the Ugandan population to aid the screening, detection, and diagnosis of tuberculosis. The team will first develop a certified training for radiographers and medical professionals on chest X-ray pattern recognition. The trained personnel will then perform chest X-ray procedures on suspected, diagnosed tuberculosis cases at selected health centers and their contacts for whom X-ray is prescribed—generating ground truth reports from their own interpretation, uploading the data and reports, and working with expert radiologists for second opinions. Suspected cases with a positive chest X-ray screening report will undergo a confirmatory test, and for diagnosed cases, data will be extracted from patient reports. The screening reports and the confirmatory tests will be used to generate labels for the chest X-ray images. From all of this, the team will created a labeled, and inclusive dataset comprising at least 2,000 unique chest X-ray images and clinical data.
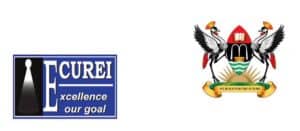