Announcing Awards for Climate Datasets: Health and Energy
16 February 2023Announcing Awards for Climate Datasets: Health and Energy
2022 Climate Awardees
Lacuna Fund is delighted to announce awards to eight teams who will create machine learning datasets in the Climate domain. Five project teams will focus on the intersection of climate and energy, studying impacts in Pakistan, Sri Lanka, Nigeria, and Mauritius. These datasets aim to improve energy systems and infrastructure for climate change mitigation and adaptation.
The remaining three teams are focused on health. Their aim is to understand climate harms to health and livelihoods, and they will be conducting their work in Kenya, Malawi, Senegal, Tanzania, Uganda, and the Philippines. These machine-learning datasets for climate and energy and climate and health energy span multiple continents, contexts, and conditions. We congratulate these teams on their awards to create open, equitable datasets in low- and middle-income countries across the globe.
We extend our deep gratitude to both of our 2022 Climate Technical Advisory Panels (TAPs) and partner reviewers for their work distilling a vibrant applicant pool and selecting a diverse portfolio of projects for funding.
Climate and Energy TAP members:
- Anders Pedersen, World Bank Group
- Daniel Dotta, State University of Campinas
- Johannes Friedrich, World Resources Institute
- Na Luo, Lawrence Berkeley National Laboratory
- Phoebe Odour, Regional Center for Mapping of Resources for Development (RCMRD)
- Ruth Schmidt, German Agency for International Cooperation (GIZ)
- Satheesh SK, Indian Institute of Science
Climate and Health TAP members:
- Alvaro Soto, Universidad Católica de Chile
- Damazo Kadengye, African Population and Health Research Center,
- Daniel Rodriguez, University of California, Berkeley
- Emmanuel Raju, University of Copenhagen
- Jeffrey Stanaway, University of Washington
- Judy Wawira Gichoya, Emory University
- Tejumade Afonja, AI Saturdays Lagos
Many thanks also to our funding partners for making these awards possible: The Rockefeller Foundation, Google.org, Wellcome Trust, and GIZ’s FAIR Forward programme on behalf of the German Federal Ministry of Economic Cooperation and Development (BMZ)
Read on to learn more about these teams and the datasets they will be building.
Climate and Health
Linking Village Level Data for Climate and Health in Philippine Cities
Contact: Pia Faustino | pia@thinkingmachin.es
Thinking Machines Data Science, in partnership with EpiMetrics, Inc., Manila Observatory, and Philippine Action for Community Led Shelter Initiatives will construct a labeled, validated, and linked dataset measuring 20 years of climate, environmental, socioeconomic, and health dimensions at the village level in 12 diverse cities in the Philippines. This granular dataset will disaggregate the disparate health risks experienced by the most vulnerable communities, especially those living in informal settlements. Data will be sourced from local and national health facilities, climate and weather institutions, open-source geospatial platforms, and previously conducted household surveys. The resulting datasets will provide policymakers, civil society, communities, governments, academia, economic enterprises, and researchers with a baseline picture of the historical and spatial connection between climate and health in the Philippines. It will also enable the development of models to anticipate and inform action to reduce and mitigate climate-sensitive health risks on the ground. The project partners bring years of expertise in climate science, health research, machine learning, and urban issues.
“Many of the causes of ill-health lie outside the health system. This grant will help us understand the effect of climate change on health inequity and potentially put climate and health on the policy agenda.”
— Dr. John Q. Wong, Founder and Senior Technical Advisor, EpiMetrics
“Our consortium is excited to leverage the Lacuna Fund award to develop a critically important dataset linking climate change and health impacts at the village level in the Philippines. We hope this dataset will help decision-makers better understand and address the disproportionate health risks faced by the most vulnerable communities in one of the most at-risk countries to climate impacts.”
— Pia Faustino, Director for Social Impact and Sustainability, Thinking Machines Data Science
INSPIRE Network: Integration and Harmonization of Health and Demographic Surveillance and Climate Data in Africa
Contact: Agnes Kiragga | akiragga@aphrc.org
The Implementation Network for Sharing Population Information from Research Entities (INSPIRE) is a collaboration between health and demographic surveillance sites (HDSS) to create a network of Longitudinal Population Studies (LPS) in Africa. INSPIRE is hosted at the African Population Health Research Center in Kenya. HDSS have provided demographic data on births, deaths, and migration in many African countries for over 20 years. Many HDSS are then used as platforms for health surveys that monitor signs, symptoms, and prevalence of different health conditions.
In this project, the team aims to link the HDSS data with remote satellite sensor climate data to understand the effect of climate change on health outcomes in African populations. Specifically, we will enhance the INSPIRE common data model with climate data generated from HDSS to predict the effect of climate change in rural and urban African communities. These openly-labeled datasets will inform community leaders, policymakers, health planners, and public health specialists on the most effective means of reducing and managing climate change in Africa.
“Climate change is the biggest global health threat of the 21st century and Africa suffers disproportionately from the effects of climate change. We are excited to receive funding from Lacuna Fund to enable the creation of open-access climate health datasets, in collaboration with data producers from African communities and health surveillance sites, to estimate climate change’s effect on African lives and inform the design of informative responsive policies.”
— Agnes Kiragga, Head of Data Science Program, African Population and Health Research Center
Tanzania Climate Sensitive Waterborne Diseases Dataset for Predictive Machine Learning
Contact: Joseph P. Telemala | josephmasamaki@gmail.com / josephmasamaki@sua.ac.tz
Advances in machine learning (ML) for healthcare applications have the potential to be an alternative and best solution to solve the problems of climate-sensitive diseases in Africa and low-income countries like Tanzania. This project will strengthen the health system in the East African region by creating a dataset that aids in the prediction and characterization of waterborne diseases as influenced by climate change. The dataset will include three waterborne diseases that are sensitive to climate change: typhoid fever, diarrhea, and amoebiasis.
Five different kinds of datasets will be used to characterize disease hotspots in five selected areas of Tanzania: Morogoro Municipal Council (MC), Singida MC, Dodoma City Council (CC), and Dar es Salaam CC (Temeke MC, Ilala MC). Datasets will be collected in five categories: (i) demographic characteristics of the waterborne diseases, (ii) locations of the toilets and quality of the toilets, (iii) management of solid wastes and dump sites, (iv) meteorological information of the hotspots, and (v) location of the water sources used by local people for daily household activities. The combination of all these datasets in tabular form will be used to train powerful machine learning algorithms to predict and characterize the outbreaks of water borne diseases in the study areas. Furthermore, the predictive models can be embedded into early warning systems to support council managers and healthcare providers to make informed decisions to control and eliminate the outbreak of waterborne diseases.
“The effects of climate change on human health are real. Outbreaks of climate-sensitive waterborne diseases in developing nations are a common disaster. If a curated dataset is made available and accessible for AI researchers to use, they can develop powerful predictive AI models that can forecast outbreaks, prevent epidemics, and save lives. With the support from Lacuna, we will develop Tanzania’s first machine learning dataset for forecasting climate-sensitive waterborne diseases”.
— Joseph P. Telemala, Sokoine University of Agriculture
Climate and Energy
Development of Emission Dataset from Abattoir Operation Facilities in Southern Parts of Nigeria
Contact: Dr. Emmanuel C. Chukwuma | ec.chukwuma@unizik.edu.ng
Preliminary survey indicates that over 400 abattoir centers (slaughterhouses) in Southern Nigeria rely heavily on wood and discarded tires for meat processing. Thick smoke is seen in the morning hours around these abattoirs as the meat processing takes place. In addition, paunch manure (rumen content) and other animal waste are seen in heaps around these abattoirs, owing to poor waste management. Additionally, in a bid to access the water needed for meat processing cost-free, more than 50% of abattoirs are located close to a flowing body of water. The visible emissions from the meat processing (thick smoke), the natural emission from the biological wastes, and disposal of wastewater to the nearby bodies of water (rivers, streams) create severe water and air pollution with further health challenges for the operators, residents, and meat buyers. This project will consider developing a climatic emission and clean energy dataset for abattoirs in the Southern part of Nigeria. The dataset will provide geospatial information, estimated air pollution, energy potential from bio-waste, and more. It is anticipated that the dataset will foster intervention in mitigating environmental pollution arising from animal slaughterhouses and serve as a clean energy source.
“The creation of open access datasets to eliminate air pollution is a responsibility for all.”
— Dr. Emmanuel C. Chukwuma, Chairperson, Alliance for Progressive and Sustainable Environment
Dataset for Energy Consumption, Needs & Forecasting Using Indigenous Tools
Contact: Zeeshan Shafiq | zeeshanshafiq@uetpeshawar.edu.pk
This project aims to deploy the utility solutions developed by Center for Intelligent Systems and Networks Research (CISNR), University of Engineering & Technology Peshawar, Khyber Pakhtunkhwa Province of Pakistan in collaboration with a local development organization, Sarhad Rural Support Program (SRSP), in selected districts of Khyber Pakhtunkhwa, Pakistan. The utility solutions developed and deployed will provide real-time monitoring, metering and faults of the electricity infrastructure at multiple levels including generation (micro-hydro power plant), distribution, and consumption. team’s dataset aims to record various parameters such as 24/7, real-time monitoring and metering, as well as faults and losses and alerts identification to concerned users and authorities. Their system will identify losses in real time and generate alerts to authorities to take necessary actions before damaging the infrastructure, and will reduce losses in neutral line due to imbalance. The dataset will be critical in assisting the administration in analyzing, evaluating, and making decisions using machine learning algorithms. The dataset will provide a variation of electricity generation and consumption parameters over time due to climate change. The AI-assisted decision will help reduce administrative and technical losses vis-à-vis energy savings.
“Pakistan is an energy insecure country. Local communities still rely on wood and fossil fuels for heating and cooking in summers and winters. Small Micro Hydro Plants (MHPs) established by Sarhad Rural Support Programme (SRSP) are one of the main sources of clean energy in these areas. To enhance reliability of electricity and ensure uninterrupted power supply by SRSP to its commercial and domestic consumers in these areas, CISNR, in partnership with SRSP, will introduce state-of-the-art utility solutions, enabling them to make the right decisions at the right time based on real-time data from multiple parameters. This system can be scaled at national and international levels in both under-developed and developing countries to reap the benefits of smart metering and control. The financial support through Lacuna Fund will ensure the development, maintenance, and utilization of this dataset for real-time monitoring, metering, predicting future demand/losses vis-à-vis energy gap, and troubleshooting in MHPs using machine learning. Since these are off-grid stations, and the only source of electricity for the local community, making these stations more reliable and providing a higher quality of service will improve the quality of life of these remote communities.”
— Prof. Dr. Gul Muhammad Khan, Director Center for Intelligent Systems and Networks Research (CISNR), University of Engineering & Technology Peshawar.
Solar Irradiance Dataset for Mauritius, Rodrigues and Agaléga
Contacts: Pro Vice-Chancellor (Academia) | pvcacd@uom.ac.mu
Mrs. Ranjani Devi Pather-Poonoosamy, Administrative Manager Pro-Vice-Chancellor’s Office (Academia) | r.poonoosamy@uom.ac.mu
In line with UN Sustainable Development Goals (SDGs) 7 and 13—Affordable and Clean Energy and Climate Action —governments worldwide, as well as in Mauritius, are taking measures to integrate renewable energy into the smart power grid. As the field of artificial intelligence (AI) evolves, the prediction of solar and/or wind patterns, with granular accuracy at different locations, can specifically help all independent power producers in managing their production and the local utility to better analyze, plan, and optimize energy deployment into the electricity grid when required.
The team’s dataset will contain periodical solar irradiance data in 12 different locations in islands of the Indian Ocean: Mauritius, Rodrigues, and Agaléga. In addition to the time series data, corresponding satellite imagery data (with resolution of at least 250m x 250m) will also be captured and compiled into another dataset. These two datasets complement each other when correlating the solar irradiance level with the degree of cloud coverage above the specific locations. Machine learning-based forecasting models can thus be implemented using patterns of both time series data and the degree of cloud cover as inputs for short- and long-term predictions of solar irradiance. Furthermore, extrapolations can be made to predict solar power generation, storage and distribution which would enhance the feeding of green energy to the grid.
“We are grateful to Lacuna Fund for giving us the opportunity to create solar irradiance datasets for Mauritius, Rodrigues, and Agaléga. We are excited about this project because the dataset will have the potential to: ensure that the country’s energy demand is increasingly met by renewable energy and provide insights for the assessment and planning of solar generated power, as well as keeping up with international commitments. Moreover, the public will benefit from a free online solar energy platform which can improve the acceptance of Solar PV technology and increase penetration of clean-technologies in the country to further reduce GHG emissions.”
— Dr. Yogesh Beeharry, Department of Electrical and Electronic Engineering, Faculty of Engineering, University of Mauritius, Réduit, Mauritius
Twenty-Month Dataset on Household Electricity Consumption and its Drivers, Collected via Meter Readings and a Longitudinal Survey
Contact: Nilusha Kapugama | nilusha@lirneasia.net
Meeting the growing demand for electricity in an environmentally sustainable manner is one of the key challenges for many developing countries. Effective demand management has been recognized as an important part of the solution, but it requires detailed data on electricity consumption, as well as consumer behavior and attitudes around electricity consumption. It is currently difficult to find such datasets that have adequate coverage, data quality, and documentation. Accessibility to such datasets is crucial in developing methods and techniques that will lead to reliable insights, better products, and policy changes that will have a transformative impact on the residential energy sector.
LIRNEasia will work with Lanka Electricity Company (LECO) to build a two-part dataset that combines electricity consumption data with corresponding drivers of electricity consumption at the household level, collected through a longitudinal survey of over 4,000 households in Sri Lanka. This high-quality, feature-rich, and representative dataset would open several opportunities for data scientists to work with experts in electricity and energy, economics, public policy, and behavioral science. These opportunities would include, among others, understanding consumer electricity consumption in depth, promoting energy efficient consumption habits through nudges and other incentives, improving national energy standards and policies, and partnering with the electricity distribution companies and policy makers on demand management initiatives.
“The lack of foreign exchange to import oil and coal for electricity generation has led to load shedding and has further exacerbated Sri Lanka’s current economic crisis. As such, the need to manage the increasing generation costs through effective demand management is crucial. The proposed dataset will enable experimentation with behavioral nudges and other solutions to manage electricity demand.”
— Helani Galpaya, CEO and Merl Chandana, Senior Researcher and project manager, LIRNEasia
Distributed energy resource management dataset
This dataset is created for distributed energy resource (DER) management in Sri Lanka. Considered DERs include microgrids, demand flexibility, and distributed solar generation. The project strives to harmonize available microgrid, utility energy meter, and distributed solar power data, and add demand flexibility to the existing data. The project team consists of multidisciplinary partners, including experts in microgrids, climate studies, demand flexibility, power distribution utility, data engineering, internet of things / edge computing, and project management. The project is designed to create a dataset that can be used to study energy and climate on multiple scales, namely: microgrids, university power distribution systems, substation service areas, and distribution utility service areas. Available data can be used to synthesize scenarios in other parts of the distribution grid to increase the granularity of studies. In future studies, additional funding can be secured to implement metering to increase available data for DER management, such as temperature sensor data in commercial buildings in the utility. The project team expects to create an atmosphere that will synergize partners’ expertise to make a lasting impact on DER development in Sri Lanka. This will assist the Sri Lankan Government in achieving its target of 70% renewable energy generation by 2030.
“Enabling prosumer participation in achieving Sri Lanka’s 70% renewable energy generation target by 2030.”
— Tharindu De Silva,Lanka Electricity Company
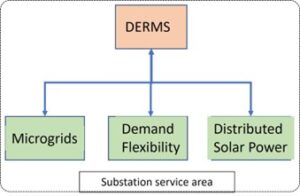