Announcing Awards for Climate and Forests Machine Learning Datasets
28 October 2024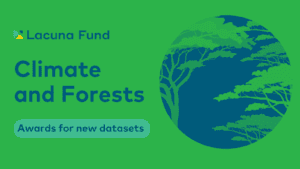
Announcing Awards for Climate and Forests Machine Learning Datasets
In 2023, Lacuna Fund launched its first Climate and Forests call for proposals within our Climate domain. Today, we are announcing 14 awardees who have received funding to create datasets within this focus. The call was made possible by funding from GIZ’s “FAIR Forward – Artificial Intelligence for all” programme on behalf of the German Federal Ministry of Economic Cooperation and Development (BMZ).
These teams will work to create machine learning datasets that advance efforts across the forestry domain to understand, mitigate, and adapt to climate change. From the Galapagos to the Congo Basin, these projects span a variety of regions and forest contexts – addressing land use change and deforestation, human-wildlife conflict, climate finance, carbon credits and more! In low- and middle-income contexts (LMICs) globally, the effective use of machine learning is hampered by a lack of ground truth data. We are excited for these Lacuna Fund grantees in Africa, Latin America, and South and Southeast Asia to help fill these gaps and develop open and accessible training and evaluation datasets.
We would like to recognize and thank our 2023 Climate and Forests Technical Advisory Panel and partner reviewers for their work distilling a vibrant applicant pool and selecting a diverse project portfolio for funding: Darlington Akogo, Jackson Efitre, Meryl Cohen, Praveen Mokkapati, Rebecca Ryakitimbo, Swetha Kolluri, Tara O’Shea, Vishwas Chitale. Learn more about the Climate and Forests TAP members.
Read on to learn more about these teams and the datasets they are building!
Lacuna Fund is a coalition of funders, data scientists, and data users including The Rockefeller Foundation, Google.org, Canada’s International Development Research Centre, German Federal Ministry for Economic Cooperation and Development (BMZ), Wellcome, Gordon and Betty Moore Foundation, Patrick J. McGovern Foundation, and Robert Wood Johnson Foundation, committed to filling data gaps and making machine learning and AI more equitable, accurate, and accessible worldwide.
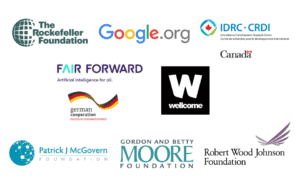
Machine learning training dataset for continental Ecuador and Galapagos
Contact: Carmen Josse | carmenjosse@ecociencia.org
This team will utilize existing data to create a new land cover classification dataset for continental Ecuador and the Galapagos islands that can be used to train multi-spectral Earth observation (EO) machine learning models. The team aims to create a dataset that consists of 30,000 points, covering a total area of 257,215.3 square kilometers. The dataset will provide information on land use/cover categories (of 20 types) and conservation status (i.e., presence or absence within protected area and/or indigenous territory), spanning the years 1985 to 2022.
This dataset will allow for a better understanding of land transformation dynamics taking place, such as forest conversion to palm oil monoculture, mangrove transformation to shrimp aquaculture, water bodies and estuarine vegetation impacted by mining, natural grasslands encroached upon by expanding forest plantation, and more. It also has the potential to identify recovery cases. For example, the Galapagos data might provide the ability to estimate if invasive species control programs have had a positive impact in vegetation regeneration or if governmental forest incentives are promoting deforestation reduction in the Ecuadorian Amazon. The land’s conservation status has the potential to predict risk of future transformation.
“Land cover/use temporal trends are essential to understand the status of the remaining natural cover and change trends. But understanding the drivers of land use/cover change with local precision has proved challenging when working with existing global-scale datasets. Lacuna Fund’s support will allow us to create a precise dataset that will elevate our ability to discriminate land use classes that are both temporally and spatially descriptive. This funding will also support an extra layer of data to support projects aimed at reducing the pressure on standing forests and help maintain the socio-natural systems that have successfully prevented conversion of forests for anthropogenic use.”
– Wagner Holguín, Coordinator of MapBiomas Ecuador, Fundación Ecociencia
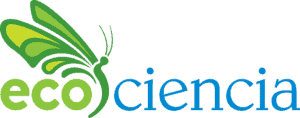
Forest carbon sequestration in the Congo Basin: combining In Situ Data and Artificial Intelligence to unlock climate finance
Contact: Kendie Kenmoe | kkenmoe@wri.org and Nancy Harris | nancy.harris@wri.org
Forests play an important role in climate change mitigation, but due to limited open forest inventory data and locally calibrated forest growth models for Central Africa, it is difficult to assess the effects of climate change on forest carbon dynamics. This, therefore, limits governments’ ability to access climate finance. To address this issue, this project will re-measure 65 field-sampled plots across forests in the Democratic Republic of Congo (DRC) and Cameroon. Data collected from these plots will be merged into a database of more than 2 million inventory plots worldwide and used to train and validate a geospatial AI-based forest growth model. Maps produced from this project will show past, current, and future dynamics of tree species distribution, species dominance and associated changes in forest carbon sequestration, and biodiversity and timber quality over time.
All field data and maps will be open access, vetted with relevant government ministries, and integrated into World Resources Institute National Forest Atlases for DRC and Cameroon. The data can be used to set credible and locally relevant forest reference levels that can unlock the “plus” of available REDD+ carbon finance – enabling sustainable forest management and an enhancement in forest carbon stocks. By increasing equitable access to data, which will increase climate financing for nature-based solutions to regions that need it most, this project will improve climate and forest outcomes.
“At a time when the Congo Basin has become the most important rainforest carbon sink, and one of the highest biodiversity hotspots in the world, understanding and tracking its dynamics is more urgent than ever if we want to achieve global 2030 climate and biodiversity goals. With support from Lacuna Fund, our project will help bring machine learning to scientists working on the ground to monitor this ecosystem. It will pilot innovative technologies to close knowledge gaps and help speed action towards better managing these rainforests, ensuring a better world for people, nature, and climate.”
— Teodyl Nkuintchua, Congo Basin Strategy and Engagement Leader and Country Manager for Republic of Congo, World Resources Institute Africa
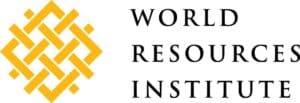
Ltome-Katip Project (“Elephant-Rat” Project): Indigenous-Led Labeling for Inclusive AI in Addressing Human-Wildlife Conflict and Climate Change
Contact: Diana Mastracci Sanchez | diana@space4innovation.com
This project is a pioneering initiative dedicated to generating Indigenous-labeled data for machine learning and AI. Led by the Namunyak Wildlife Conservancy, Space4Innovation, and the Indigenous Musap Biological Station – and spearheaded by the Shuar Nation in the Ecuadorian Amazon and the Samburu tribe in northern Kenya – this project addresses the urgent need for Indigenous-labeled data for machine learning. In collaboration with the Rochester Institute of Technology and the GEO Indigenous Alliance, the project focuses on regions heavily impacted by climate change, namely the Amazon jungle and northern Kenya. The team’s mission is to revolutionize approaches to human-wildlife conflict and climate resilience.
By actively involving Indigenous communities in data collection, labeling, and map development, the team will bridge critical gaps in understanding climate change, human-wildlife conflict, and afforestation and deforestation. This collaboration not only safeguards invaluable biodiversity and preserves Indigenous identity but also empowers communities, ensuring culturally relevant and effective solutions. Through the integration of Indigenous knowledge and cutting-edge technology, the team will be poised to make a tangible difference in the lives of both people and wildlife in these vital regions – while reducing bias and uncertainty in AI models.
“Through the integration of Indigenous knowledge and cutting-edge technology, the Ltome-Katip project not only addresses pressing environmental and technical machine learning challenges but empowers Indigenous communities to lead the way in conservation efforts. This initiative, in collaboration with the Rochester Institute and Space4Innovation, represents a true partnership between the Shuar tribe in the Ecuadorian Amazon and the Samburu tribe in northern Kenya, showcasing the power of collaboration and Indigenous knowledge in safeguarding our planet’s biodiversity.”
— Mario Vargas Shakaim (Shuar), Ltome-Katip Indigenous-Led Monitoring Project GEO Indigenous Alliance
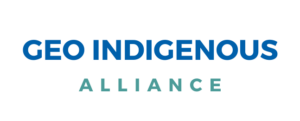
Africa Biomass Dataset: In-situ biomass measurements collected in three mangroves in Côte d’Ivoire
Contact: Therence Temfack | therence.temfack@data354.co
Mangroves play a vital role in climate resilience – especially for carbon sequestration. This project will collect data from field missions across various mangroves in Côte d’Ivoire, and label them with corresponding biomass and carbon stocks. These ground truth data will be associated with corresponding satellite images to develop an AI model capable of estimating biomass and carbon stock above and below ground remotely. This project will contribute to the protection and expansion of these forests by facilitating access to carbon credits and other payments for environmental services.
“As far as monitoring and calculating forest carbon stocks are concerned, there is still a major technological bottleneck blocking the large-scale development of forest carbon credits in Côte d’Ivoire, which needs to be overcome quickly. Remote sensing is a useful tool in the forest monitoring process; further studies should be carried out to enable it to meet the need for assessment on a smaller scale and to measure biomass directly. In this way, we can help decision-makers orient their conservation policies, but also support the process of rewarding small players, such as agricultural producers involved in agroforestry, private forestry, etc. Our aim, with the funds raised, is to provide practical field data to better train artificial intelligence algorithms for dynamic mapping of forest biomass and mangroves.”
— Abraham Bio, Actum Dev General Manager
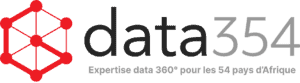
Reference Data Collection for Improving Land Use Change Mapping in Ghana
Contact: Foster Mensah | fkmawusi@gmail.com
This dataset will allow for the training and validation of machine learning-based applications to improve the mapping of cocoa and forests, as well as other important land uses and land covers in the area. The team will be undertaking an extensive field data collection campaign in the cocoa-growing southern region of Ghana. Cocoa fields cannot easily be identified for annotation, even in high-resolution imagery; instead, they require ground truthing for reliable reference datasets to be created. A lack of openly available reference data limits monitoring capability for this and other key drivers of deforestation in West Africa.
Led by the Centre for Remote Sensing and Geographic Services (CERSGIS) and supported by researchers from the International Crops Research Institute for the Semi-Arid Tropics (ICRISAT), Boston University, and the University of Alabama in Huntsville through SERVIR West Africa and the SERVIR Global network, this work will leverage strong local connections to engage youth and community members in data collection and capacity-building opportunities. With the support of the Forest Data Partnership, this data will be instrumental in reducing commodity-driven deforestation and accelerating the restoration of degraded lands.
“We are extremely grateful to Lacuna Fund and are delighted that our reference data collection effort will contribute immensely to an evolving data ecosystem to set up scalable machine learning applications for monitoring land-use change in cocoa-forest mosaic landscapes. Led by the Centre for Remote Sensing and Geographic Services (CERSGIS) and supported by researchers from the Centre for Climate Change and Sustainability Studies University of Ghana, International Crops Research Institute for the Semi-Arid Tropics (ICRISAT), Boston University, and the University of Alabama in Huntsville through SERVIR West Africa and the SERVIR Global network, this work will leverage the local knowledge of youth and community members for science-based participatory data collection. This data will provide critical input for enabling the transformation of commodity-driven supply chain traceability and commercial opportunities for ecosystem sustainability.
— Foster Mensah Center for Remote Sensing and Geographic Information Services University of Ghana
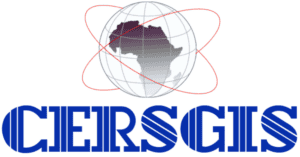
An integrated dataset combining remote sensing, ground measurements and weather data for improved reforestation monitoring
Contact: Ciira Maina | ciira.maina@dkut.ac.ke
This team will collect a high-resolution dataset of drone imagery, ground truth tree parameter measurements, and aggregate weather data from a large, reforested area in Kenya. These data will enable the development of tools that can rapidly assess the success of reforestation efforts by enabling counting of trees, estimation of trees’ biophysical parameters from drone images, and prediction of tree growth in relation to tree species and weather variables.
“Machine learning based tools have the ability to help monitor reforestation efforts in Africa and we believe the dataset we will collect will contribute to these efforts. We also hope the dataset will trigger new methodological developments in the important area of environmental conservation.’”
— Prof. Ciira Maina, Centre for Data Science and Artificial Intelligence, Dedan Kimathi University of Technology.

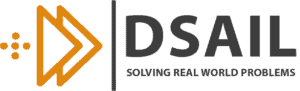
Datasets for artificial intelligence-based solutions in predicting land use/cover changes in Uganda
Contact: Sarah Akello | sarahakellok1@gmail.com
Deforestation is a significant contributor to changes in land use/cover. This challenge is intensified by agriculture’s extreme vulnerability to climate change. Stakeholders are increasingly turning towards artificial intelligence to support decision-making in natural resource management. This project, therefore, aims to generate accessible, quality satellite imagery datasets and National Forestry Inventory datasets for Uganda. These datasets will be utilized to predict land cover types or changes for informed decision-making in natural resource management. This research will utilize Sentinel 2 and tree cover satellite imagery datasets and augment them with the inclusion of inventory datasets. In partnership with the Uganda National Forestry Authority (NFA), the project team aims to curate Sentinel 2 and Hansen tree cover image datasets. A biomass mean stock per hectare dataset for each vegetation type will also be acquired. Annotation procedures of the different datasets will be further conducted to deliver on each intended machine learning task.
“With funding from Lacuna Fund, satellite image and forest inventory datasets will help to develop machine learning models for prediction of land cover types or changes for informed decision-making in natural resource management. Under joint research from Makerere College of Agriculture and Environment Studies and Makerere AI Health Lab, and in partnership with the National Forestry Authority, Uganda, our land use/cover change datasets will improve our understanding of the relationship between land cover/land use change and climate change. It will also be useful in informing practice and policy on how best to manage various potential scenarios.
— Dr. Sarah Akello, College of Agriculture and Environmental Sciences, Makerere University
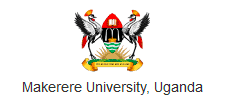
African Trees for Climate Resilience: A Comprehensive Bioinformatics Approach
Contact: Guy Franklin Midgley | gfmidgley@sun.ac.za
Trees are structurally important components of southern African forests, thickets, and savannas. They support millions of African livelihoods now threatened by climate change. This project will develop an extensive bioinformatics resource that leverages tree species’ medicinal, food provision, and other trait data, together with southern African trees’ climate relationships and growth characteristics for climate adaptation and mitigation planning. Tree species data – including from the international Global Biodiversity Information Facility (GBIF) database and Botanical Information and Ecology Network (BIEN) programme – as well as land-use, climate, geological, and soil data, will be collated to define southern African tree species’ biogeoclimatic niches.
The primary application of this work will include identifying indigenous species that can enhance ecological resilience by mapping adaptation and mitigation opportunities and assessing climate risks to African trees. Existing cutting-edge functional niche modeling will allow for the identification of areas for optimal use of African trees based on the results of tree growth performance. This will promote the use of indigenous trees for reforestation, regenerative agriculture, ecological restoration, human health and livelihood support, and urban afforestation programs to adapt to and mitigate the impacts of climate change.
“We are excited that Lacuna Fund has provided Stellenbosch, and our team, with the opportunity to utilize these datasets to enable researchers to model the impacts of indigenous trees for adaptation and mitigation to climate change in southern Africa. This information is of the utmost importance in ensuring the continent can build resilience against the rapid unfolding of climate change which is affecting livelihoods and demanding that we find solutions.”
— Professor Guy Midgley, Acting Director at School for Climate Studies, Stellenbosch University
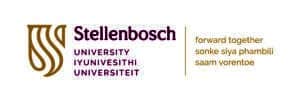
Blue carbon in West Africa: mobilizing datasets to better understand mangrove health in relationship to climate change
Contact: Romain Glèlè Kakaï | glele.romain@gmail.com or romain.glelekakai@fsa.uac.bj
Mangroves are valuable coastal ecosystems, providing diverse services to humans and playing a critical role in the global strategy to mitigate impacts of climate change. Regular monitoring of their conditions is essential to provide evidence that supports timely decisions for their management. Yet, there is a critical lack of comprehensive ground truth and long-term data on mangrove biodiversity, structure, environmental conditions, socio-cultural contexts, and ecosystem services that can help identify key factors contributing to mangrove health, feed AI and ML platforms that generate valuable knowledge, and inform effective conservation strategies. The “Benin Mangroves Open Data (BeMOD)” project will generate 500 annotated images, 1,920 observations on water quality, 1,920 observations on soil characteristics, 10,800 climate observations, forest inventories data (tree diversity, canopy height and cover, tree diameter, total height, biomass, etc.) from 600 plots, and data on socio-cultural contexts (e.g., uses, activities) of mangroves in 20 sites in Benin. The project will cover Ramsar sites 1017 and 1018 which are areas where mangroves are confined in Benin. This dataset will be freely available on diverse open data platforms but will also be used to write scientific articles that contribute to improve current knowledge on mangroves in West-Africa.
“Funding from Lacuna Fund for our project will contribute to generating one of the most comprehensive open datasets for mangroves in West Africa, thus enhancing knowledge sharing and uptake locally, regionally, and globally on this critical ecosystem for their sustainable management.”
— Romain Glèlè Kakaï, Laboratoire de Biomathématiques et d’Estimations Forestières, Faculty of Agronomic Sciences, University of Abomey-Calavi, Republic of Benin
Advancing oil palm mapping in Indonesia with social forestry and machine learning
Contact: Peter Cutter | peter.cutter@recoftc.org
This project aims to improve understanding and mitigation of the impacts of oil palm cultivation on forests and climate change, support sustainable forest management practices, and empower local communities in forest resource management. The project will create open and accessible training and evaluation datasets for machine learning applications focused on forest cover and change in Indonesia. The datasets will contain collections of polygons of uniform land cover, with labels indicating land cover type and, possibly, information on land cover change over time. To label land cover patches, the team will review high-resolution satellite imagery facilitated by Collect Earth Online (CEO) and augmented with field visits to difficult-to-classify areas. Intended beneficiaries of these outputs are local communities engaged in community forestry in Indonesia, as well as researchers, policymakers, and civil society organizations.
“Communities in the areas targeted by this project are challenged when it comes to mapping the rapidly changing forest landscapes on which they depend. The datasets this project generates will provide valuable insight into these dynamic patterns of change.”
— Gamma Galudra, Director, Indonesia Country Office, RECOFTC
Inclusive Digital Monitoring System in the Eastern Himalayas
Contact: Michael Anthony | michael@vertify.earth
The Indian Himalayas are a critical hotspot for biodiversity and forest conservation, facing unique challenges of deforestation due to population pressure, agricultural expansion, and infrastructure development. In addition, the Himalayas play a vital role in regulating climate, water resources, and sustaining livelihoods for millions. Conservation organizations such as Balipara Foundation are seeking to monitor the impact of conservation efforts and to map areas suitable for new restoration interventions. and to consistently monitor the impact of conservation efforts.
In this project, Vertify.earth and Alsisar Impact, both from Mumbai, will develop a monitoring, reporting, and verification tool (“MRV”) that measures key trends in degradation and conservation of Himalayan ecosystems. The tool will rely both on geospatial data (from optical, radar, and LIDAR satellites) as well as on-ground data that is collected by young villagers from Indigenous Hubs that local partners have set up. Youth members from these hubs, guided by Balipara’s science team and local universities, will lead the ground data collection efforts. Their focus will be on gathering crucial biodiversity and forest parameters to calibrate geospatial data. This dataset will be processed, stored, and published following the SpatioTemporal Asset Catalog (STAC) principles. Existing data will also be standardized to adhere to these standards.
“Lacuna Fund will be instrumental in supporting the development of an MRV system that will enable us to participatorily map the health of forests in the biodiverse Eastern Himalayan region with indigenous communities, facilitating targeted interventions for climate resilience through forest management – impacting the millions of people that depend on forests for their lives and livelihoods in the Eastern Himalayas.”
— Michael Dawson, Rural Futures – Anthropological Visioner, Balipara Foundation
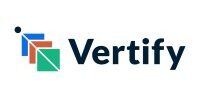
Agrof4resilience: data-enabled climate shock absorbance and ecosystem resilience through agroforestry
Contact: Dr. Elfaith M. Abdel-Rahman | eabdel-Rahman@icipe.org
The International Center of Insect Physiology and Ecology (ICIPE), through generous support from Lacuna Fund, is pleased to announce a significant initiative aimed at enhancing food security, nutrition, and environmental sustainability in Kenya. Agroforestry systems (AFSs) play a vital role in achieving these goals by mitigating climate change impacts and creating financial benefits through timber and carbon markets. However, there is a pressing need for precise information regarding the location and configuration of AFSs across Kenya, which has posed challenges for carbon market evaluations, spatial sampling, and mapping exercises. To address this, this project will collect and publish extensive open-access data on AFSs. The team will gather data from a minimum of 20,000 land use points spanning four transects across Kenya’s agroecological zones, encompassing 35 out of 47 counties. Within a 200-hectare sample area, we will conduct thorough tree species identification, measuring height and diameter at breast height (DBH) for a total of 15,000 individual plants. Each plant will be sampled, photographed, and geotagged.
In order to support capacity building, the team plans to train at least 20 individuals in tree identification and data collection across the four transects. Additionally, at least one representative from each county government (at least 37 individuals) will receive training on accessing and utilizing this valuable dataset. The project will include community engagement by facilitating 40 focused group discussions, with a minimum of eight participants in each (including 50% women). These discussions will capture data on AFS preferences, gender roles, and the involvement of youth in AFSs, ensuring clear and interoperable labeling of this information.
The team’s goal is to create a robust, machine-learning-ready dataset that will empower further analytics and modeling using machine-learning algorithms. This data will be instrumental in optimizing AFSs, enhancing species identification, and valuing carbon sequestration. Ultimately, we will collaborate closely with the Kenyan government through the Kenya Forestry and Research Institute (KEFRI), as well as with Strathmore University in Kenya, to ensure the responsible and sustainable management of AFSs for the benefit of all.
“The Lacuna-funded Agro4Resilience Project offers Kenyan farmers and decision makers a pathway for informed decision-making that supports tree cover increase. It will also improve agricultural productivity and mitigate climate change effects through ecosystem services.”
— David Makori, International Centre of Insect Physiology and Ecology (ICIPE)
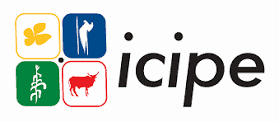
Quantifying Colombian mangroves aboveground biomass and carbon content
Contact: Maria Cuevas-Gonzalez | maria.cuevas@cttc.es
This project aims to develop a comprehensive dataset consisting of field, Unmanned Aerial Vehicle (UAV), and remote sensing data for accurate estimation of above-ground biomass (AGB) and above-ground carbon (AGC) in the Caribbean mangrove ecosystem in Colombia. The pilot area is in the Via Parque Isla de Salamanca National Natural Park (VIPIS), located in the Magdalena department in the Colombian Caribbean. VIPIS is a protected area that is part of the Ciénaga Grande de Santa Marta (CGSM), which was declared a Ramsar Site of worldwide importance in 1998 and a Biosphere Reserve by UNESCO in 2000 (Moreno-Bejarano & Alvarez-Leon, 2003; UNESCO, 2001). VIPIS is characterized by the presence of extensive mangrove forests that serve as habitat for multiple species and provide valuable ecosystem services. However, VIPIS has been affected in recent decades by human intervention, leading to deforestation, which reduces its capacity to capture and store carbon and modifies the ecological regime of the ecosystem. Although some studies have been able to estimate AGB and AGC at a global level, including within the CGSM, the modeling needs to be implemented with greater precision and cost-effectiveness.
This dataset will incorporate existing field data as well as new field and UAV data specifically collected for this project, focusing on the structural parameters of trees within sample plots distributed across the pilot area. The dataset will serve as input for machine learning applications, enabling the development of robust models for AGB and AGC estimation with satellite imagery. By combining field data with remote sensing information, this study aims to enhance the accuracy and efficiency of AGB and AGC estimation within the study area. Additionally, statistical analysis will be conducted to assess the relationships between the parameters measured in the field and those obtained through remote sensing techniques. The dataset will facilitate accurate AGB and AGC estimation in Colombian Caribbean mangroves, enabling a better understanding of carbon storage and supporting conservation and management efforts. It will also provide a valuable resource for future research and monitoring activities related to these important coastal ecosystems.
“The dataset compiled during the project will facilitate accurate AGB and AGC estimation in Colombian Caribbean mangroves, enabling better understanding of carbon storage and supporting conservation and management efforts. It will also provide a valuable resource for future research and monitoring activities related to these important coastal ecosystems. Furthermore, the methodology developed in this project might even be applied beyond the Colombian Caribbean to other mangrove ecosystems with similar structures.”
— María Cuevas-González, Geomatics Research Unit, Centre Tecnològic de Telecomunicacions de Catalunya (CTTC)
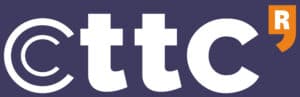
Phenological Dataset for Ecological Forecasting (PheDEF Project)
Contact: Bismark Ofosu-Bamfo | bismark.ofosu-bamfo@uenr.edu.gh
The health of tropical forest ecosystems faces pressure from climate change, threatening the sustainable supply of leaves, flowers, and fruits which provide important resources for wildlife, domestic animals, and human settlements. This project aims to monitor and provide data on the timing of plant life cycle events (phenology) in Ghana, West Africa from multiple sources to track the availability of plant resources and how this is impacted by climate variability. This dataset builds on previous data collection on the phenology of the woody vine plants, lianas, from ground observations in two tropical forest ecosystems (a moist semi-deciduous and a dry semi-deciduous forest) and will be expanded to include tree phenology, phenology of species based on traditional ecological knowledge of local communities, use of trail cameras (phenocams), and satellite images.
Phenology data from multiple sources would be combined with local climate data (rainfall, temperature, relative humidity and solar radiation) to create a harmonized machine learning analysis-ready dataset that can be used to predict phenology at community and landscape scales. This dataset will enhance the representation of tropical African forests in phenology research and contribute meaningful data from tropical African forests for machine learning applications in climate, forests, and biodiversity conservation. Machine learning has achieved a level of stability and effectiveness, however, systematically curated data is still required for good ecological forecasting using ML methods.
“The threat of climate change on forest health affects leaf, flower, and fruit resource availability, but we cannot be prepared unless we have adequate data with predictive power. We have an incredible opportunity to create a harmonized machine-learning-ready dataset for ecological forecasting with the support of Lacuna Fund. Such a forecast could provide the basis for an early warning system that will help communities and resource managers better plan and prepare for climate change impact on forest resources.”
— Bismark Ofosu-Bamfo, Department of Biological Sciences, University of Energy and Natural Resources
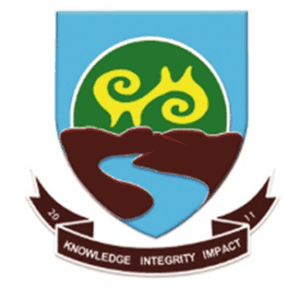