Five New Machine Learning Datasets in Agriculture, Health, and Language Domains
28 August 2024Five New Machine Learning Datasets in Agriculture, Health, and Language Domains
Today, we are excited to announce five recently published datasets for training artificial intelligence in the domains of Agriculture, Health, and Natural Language Processing (NLP). These datasets harness the power of AI to address urgent social and economic problems in Africa and Latin America.
Learn more about these datasets and how to access them below!
Lacuna Fund is a coalition of funders, data scientists, and data users including The Rockefeller Foundation, Google.org, Canada’s International Development Research Centre, German Federal Ministry for Economic Cooperation and Development (BMZ), Wellcome, Gordon and Betty Moore Foundation, Patrick J. McGovern Foundation, and Robert Wood Johnson Foundation, committed to filling data gaps and making machine learning and AI more equitable, accurate, and accessible worldwide.
We extend our deep gratitude to our funders who make the creation of these datasets possible.
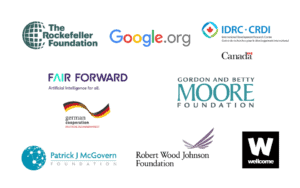
Agriculture
A region-wide, multi-year set of crop field boundary labels for Africa
Contacts:
- Mary Dziedzorm Afenyo | Farmerline | mary@farmerline.co
- Lyndon Estes | Clark University | lestes@clarku.edu
- Primož Kovačič | Spatial Collective | primoz@spatialcollective.com
This dataset provides continent-wide crop field labels for Africa, improving the availability and use of crop field boundary (parcel) maps. It contains 42,403 annotated geospatial polygons indicating the boundaries of individual crop fields spanning the years 2017-2023. These annotations, done by the project team, were created in combination with existing satellite imagery for 33,746 unique field boundary sites. The sites were defined as unique spatial locations of approximately 550 meters by 550 meters, overlaid on the satellite images.
The outputs from this project include GeoParquet field boundary files; a CSV file with ID, name, coordinates, date, and quality metrics; digitized planet image chips for each site; a Jupyter notebook to filter the quality metric catalog and create rasterized labels; a CSV file with an example filtered catalog from the notebook; and a set of example rasterized labels from the notebook. This can be used for field labeling, training models to map agricultural fields over large areas and multiple years.
This dataset can be used in a variety of ways to train and assess machine learning models for agricultural applications. Models could learn to distinguish between boundaries and the interiors of fields with boundary-aware semantic segmentation. It might also be used to create binary crop and non-crop labels. Finally, the full catalog can be used to test the impact of label quality on overall model performance.
Authors and Affiliations:
- Authors: Wussah, A., Afenyo, M., Osei , A.K., Gathigi, M., Kovačič, P., Muhando, J., Addai, F., Akakpo, E.S., Allotey, M., Amkoya, P., Amponsem, E., Dadon, K.D., Gyan, V., Harrison X.G., Heltzel, E., Juma, C., Mdawida, R., Miroyo, A., Mucha, J., Mugami, J., Mwawaza, F., Nyarko, D., Oduor, P., Ohemeng, K., Segbefia, S.I.D., Tumbula, T., Wambua, F., Yeboah, F., Estes, L.D., 2024.
Dataset:
- Zenodo: https://zenodo.org/records/11060871
- Github: https://github.com/agroimpacts/lacunalabels
- AWS Open Data Registry: https://registry.opendata.aws/africa-field-boundary-labels/
Health
Childhood Malnutrition in Chile
Contact: Maria Paz Hermosilla | goblab@uai.cl
This data repository will evaluate factors that contribute to child malnutrition in Chile and childrens’ nutritional status, as well as the associated costs. The focus at this stage is on estimating health costs associated with child malnutrition and identifying biopsychosocial determinants that lead to it. Before the beginning of this project, there was no integrated repository to inform policies around this issue in Chile.
There are a total of more than 1.4 billion records in this repository, classified by data source and by specific period. The longitudinal database of children under 18 years old contains information on health, family, school, social and cultural factors, health-related spending, and other related data such as information about family members that may be relevant for future studies. Most of the data comes from 2015-2022, although some of the databases include older data (e.g., births from 1992-2022; hospital discharges from 2001-2022).
Authors and Affiliations:
- Ministry of Health, Chile
- GobLab, School of Government, Adolfo Ibañez University, Chile
- FONASA (Public health insurance agency)
- Health Superintendency, JUNAEB (national school aid and scholarship board).
Dataset: Given the sensitive nature of the data contained in this repository, those interested can visit the project website here for controlled access for relevant awarded research projects: https://goblab.uai.cl/proyecto-reduccion-de-la-malnutricion-infantil-en-chile/.
Lacuna Malaria Datasets
Contact: Rose Nakasi | g.nakasi.rose@gmail.com or rose.nakasi@mak.ac.ug
This dataset will aid in the diagnosis of malaria. The dataset contains annotated images of blood samples collected in Uganda and Ghana with objects of interest, including parasites and white blood cells. It significantly increases the number of available microscopy images — including metadata — by 6,000 thick blood slides and 2,000 thin blood slides for use in object detection research and other areas of inquiry.
This work is a product of a collaboration between Makerere Artificial Intelligence Lab and minoHealth. The team at Makerere University collected 4,000 images, including 1,000 thin blood slides (100% annotated), and 3,000 thick blood slides (82% annotated). The minoHealth team collected an additional 1,000 thin blood slides and 3,000 thick blood slides. The annotations include bounding boxes showing malaria parasites and white blood cells for thick blood smear images and malaria parasites, parasite type (Trophozoite or Gametocyte), and parasitized cells for thin blood smear images. Some images also include data on the physical slide from which the image was captured, such as the stage micrometer readings of the microscope, and the microscope objective settings used to capture the image.
Authors and Affiliations:
- Makerere Artificial Intelligence Lab
- minoHealth
Dataset: https://doi.org/10.7910/DVN/VEADSE
Language
BIG-C: A Multimodal Multi-Purpose Dataset for Bemba
Contact: Claytone Sikasote | claytonsikasote@gmail.com
The BIG-C (Bemba Image Grounded Conversations) dataset is comprised of multi-turn dialogues between Bemba speakers grounded on images, transcribed and translated to English. Specifically, there are over 92,000 sentences, amounting to over 180 hours of speech data with corresponding Bemba transcriptions and English translations. Bemba is the most widely spoken language in Zambia but a lack of linguistic data resources has constrained advancements and applications in language technologies and language processing research. This project has built the first ever large-scale multimodal dataset for Bemba to use for speech recognition, machine translation, speech translation, language modeling, multimodal translation systems, and grounded learning based on images. It is a crucial resource for research and development of language technologies for Bemba languages.
By making the dataset available to the public and research community, this project will foster research and encourage collaboration across the language, speech, and vision communities, especially for traditionally under-resourced languages.
Authors and Affiliations:
- Claytone Sikasote, University of Zambia, Zambia
- Eunice Mukonde – Mulenga, University of Zambia, Zambia
- Md Mahfuz Ibn Alam, George Mason University, USA
- Antonios Anastasopoulos, George Mason University, USA
Dataset: https://github.com/csikasote/bigc
Publication: https://aclanthology.org/2023.acl-long.115
KALLAAMA
Contact: Aminata Ndiaye | amina.ndiaye@jokalante.com and Elodie Gauthier | elodie.gauthier@orange.com
This dataset will strengthen natural language processing resources for Wolof, Pulaar, and Serer, the three most widely spoken languages in Senegal.
Although datasets exist in Wolof, there is a lack of data for Pulaar and Serer. This project has played a crucial role in filling this gap. This dataset’s repository of transcribed speech includes over 55 hours (12 files) of transcribed speech in Wolof, 38 hours (105 files) in Serer, and 31 hours (83 files) in Pulaar. The repository also includes over 12 hours of verified recordings in each language, textual data containing over 947,000 words in Wolof, and 593,000 in Pulaar. It also includes a pronunciation lexicon of over 54,000 phonetized entries in Wolof.
This dataset can be used to solve tasks including speech-to-text, question answering, and language learning, and can help fine-tune multilingual models. The data can also be used to develop speech modeling, automatic response modeling, local-language speech recognition, transcription systems, and personal assistants capable of answering questions relating to agricultural advisories for smallholder farmers.
Authors and Affiliations:
- Project Leader: Aminata Ndiaye Diallo (Jokalante, Dakar, Senegal)
- Stakeholders: Elodie Gauthier (Orange Innovation, Lannion, France), Abdoulaye Guissé (Ecole Polytechnique de Thiès, Senegal)
- Intern: Boubacar Diallo (Assane Seck University , Ziguinchor, Senegal) – Collection of textual dataset
- Trainees: Maimouna Diallo (Cheikh Anta Diop University , Dakar, Senegal) – Wolof transcription, Houleye Amadou Kane (Cheikh Anta Diop University , Dakar, Senegal) – Pulaar transcription, Fatou Diouf (Cheikh Anta Diop University, Dakar, Senegal): – Serer transcription
Dataset:
- Github: https://github.com/gauthelo/kallaama-speech-dataset
- OpenSLR: https://www.openslr.org/151/
- Zenodo : https://zenodo.org/records/10892569