Announcing Awards for African Language Datasets — 2021 NLP Awardees
19 October 2022Today, we are delighted to announce awards to 10 teams to create or expand machine learning datasets for low-resourced African languages. These projects feature languages across the continent of Africa and will enable a range of use cases, from providing citizens’ access to news and information in their native languages, to developing models for the anonymization of data. Datasets for widely spoken Nigerian languages, indigenous Kenyan languages, and the Bantu language Emakhuwa in Mozambique will extend the benefits of language technologies to millions of Africans.
We extend our deep gratitude to our 2021 Language Technical Advisory Panel and partner reviewers for their work distilling a vibrant applicant pool and selecting a diverse portfolio of projects for funding. Technical Advisory Panel members include:
- EM Lewis-Jong, Mozilla Foundation
- Clara Rivera, Google
- Kọ́lá Túbọ̀sún, Yorùbá Names Project
- Christian Resch, Deutsche Gesellschaft für International Zusammenarbeit, FAIR Forward
- Michael Melese, Addis Ababa University
- Joyce Nakatumba-Nabende, Makerere University
- Ignatius Ezeani, Lancaster University
Many thanks also to our funding partners for making these awards possible: The Rockefeller Foundation, Google.org, Canada’s International Development Research Centre, and GIZ on behalf of the German Federal Ministry for Economic Cooperation and Development.
Congratulations to the teams selected to create or expand datasets for African languages!
- Naija Voices: Curation of Speech-Text Corpora for Igbo, Hausa and Yoruba Languages
- United States International University-Africa: Building Parallel Corpora for Three of Kenya’s Indigenous Languages and Swahili
- Centro de Linguística da Universidade do Porto, Universidade Lúrio, and Laboratory of Artificial Intelligence and Computer Science at the University of Porto (LIACC): Expanding a parallel corpus of Portuguese and the Bantu language Emakhuwa of Mozambique
- Marconi AI Lab, Makerere AI Lab, and CLEAR Global: Datasets marking Personal Identifiable Information (PII) for Sub-Saharan Africa Languages
- MasaKhane NLU: Conversational AI and Benchmark Datasets for African Languages
- Bahir Dar University—Bahir Dar, Ethiopia; Bayero University—Kano, Nigeria; Rewire; Masakhane; LT Group—Universität Hamburg; and Laboratory of Artificial Intelligence and Decision Support (LIAAD): AfriHate: Hate and Offensive Speech Dataset for African Languages
- Igbo API: A Multi-Dialectal Lexical Database for Igbo
- Addis Ababa University–School of Information Science, College of Natural and Computational Sciences: Development of Speech Corpora for Six Ethiopian Languages
- Jokalante, Orange France, University of Dakar, and ESP (ecole supérieur polytechnique): KALLAAMA
- MasakhaneDAMT: African language translation dataset for domain adaptation
Read on to learn more about these teams and the datasets they will be building.
NaijaVoices: Curation of Speech-Text Corpora for Igbo, Hausa and Yoruba Languages
NaijaVoices will provide digitization support for low-resourced African languages through the development of 500-hour speech datasets in three Nigerian languages: Igbo, Yoruba and Hausa.
Given the lack of audio and text datasets in Nigeria and across Africa, a large portion of these populations are being excluded from the benefits of new technological advancements in machine learning and artificial intelligence. This project was conceived to fill this gap.
This large volume of audio datasets will enable further research in machine learning and spur development of artificial-intelligence-related technologies in the areas of education, health, agriculture, health, engineering, and finance. This project could also assist in forging stronger national unities and inter-ethnic bonds in Nigeria through the development of speech translation apps and gadgets in these languages.
“Existing speech recognition services are not available in many African languages (current voice assistants like Amazon’s Alexa, Apple’s Siri, and Google’s Home do not support a single African language), so the speakers of these languages are excluded from the benefits of voice-enabled technologies. This dataset will no doubt pave the way for speech technologies—such as speech-to-text, text-to-speech, speech translation, and acoustic modeling—for these African languages, which hitherto had few or no public datasets.”
– Chris Emezue, NaijaVoices
Building Parallel Corpora for Three of Kenya’s Indigenous Languages and Swahili
Government communication is critical for the safety and well-being of citizens. In Kenya, this communication is in English and Swahili, but many people in rural communities have a limited understanding of these official languages. This leads to reduced access to essential information during times of crisis and creates room for dangerous rumors and misinformation.
The United States International University-Africa, in collaboration with Maseno University, Kabarak University, and University of Florida, proposes machine translation as a possible solution to this problem, where communications in Swahili are automatically translated to the relevant indigenous languages. Lacuna Fund will help this team to take the first step in that direction by building parallel corpora for Swahili and Kitaita, Kalejin and Dholuo. They aim to collect a total of 900,000 sentence pairs by crowdsourcing translations. The team will take advantage of the significant amount of Swahili data available and recruit translators from the many people in Kenya who are fluent in both their mother tongue and Swahili. The data generated will serve as a starting point for longer term machine translation work in Kenya for the three languages and beyond.
“Natural Language Processing (NLP) for low-resource languages cannot be rushed. It is a marathon, not a sprint, and involving communities on a large scale is key. As the African proverb says: if you want to go fast, go alone. If you want to go far, go together. We aim to go far.”
– Audrey Mbogho, United States International University-Africa
Expanding a Parallel Corpus of Portuguese and the Bantu Language Emakhuwa of Mozambique
Centro de Linguística da Universidade do Porto, Universidade Lúrio, and the Laboratory of Artificial Intelligence and Computer Science at the University of Porto (LIACC) aim to expand a parallel corpus for Machine Translation (MT) to/from Emakhuwa. Emakhuwa is widely spoken in northern and central Mozambique by approximately 7 million speakers—10 percent more than the country’s official language of Portuguese – but has relatively few resources dedicated to it. As a result, acquiring text data to train Machine Translation models in Emakhuwa is very challenging.
With support from Lacuna Fund, the team will generate translation memories based on the corpus containing Voice of America (VOA) news published between 2001 and 2021. The dataset will be released publicly and will also contain annotation for Named Entities (PER, LOC, ORG, DATE) and news classifications labels (politics, economy, culture, sports, and world).
“Africans should not lose hope of one day accessing education and information in their mother tongues. In fact, it has been proven that instruction given in the mother tongue can have a significant effect on reducing the illiteracy rates that still prevail in the continent. Technology can help to shorten this gap in native language usage, especially now that the younger population has increased access to technology. The demand for the development of language tools in Africa is increasing, as is the need for resources to build such tools. Lacuna Fund gave us a unique opportunity to contribute to resource creation for the Bantu Mozambican language Emakhuwa, the most widely spoken language in Mozambique.”
– Felermino Ali, LIACC
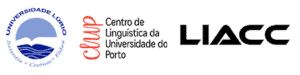
Datasets Marking Personal Identifiable Information (PII) for Sub-Saharan Africa Languages
This project is a collaboration between Marconi AI Lab, Makerere AI Lab and CLEAR Global. The aim of this project is to create voice and text datasets for four major Sub-Saharan African (SSA) languages in East and West Africa—specifically, Uganda and Nigeria. The datasets will be labeled for Personal Identifiable Information (PII), according to best practices and standards. Part of this work will involve establishing guidelines for PII tagging for the languages that could serve as a rubric for other low-resourced languages.
For each of the four languages, the team will compile labeled text datasets that include PII. The datasets will be sufficiently large to provide around 1,000 instances of key classes within at least 3,000 sentences collected for each language. These datasets will be used to develop PII tagging models which will serve as a core component for the anonymization of data. Reliably removing PII from existing datasets will allow those datasets to be released for training Natural Language Processing (NLP) models. The resulting datasets will make it possible to address issues of PII in downstream voice technologies such as automatic speech recognition (ASR), natural language understanding (NLU), and machine translation (MT) applications for these languages.
“All major global technology companies (Google, Facebook, Microsoft, etc.) have initiatives to create datasets for PII sanitization for major languages, in the hope of unlocking more training data for their products. Without similar tools for low-resourced languages, the digital divide will grow for communities such as those in SSA, that primarily communicate in non-majority, native languages. To address this challenge, there is a need to build NLP text and speech technology for SSA, starting with the creation of high-quality, open, PII-clean, and bias-free datasets.”
– Dr. Andrew Katumba, Marconi AI Lab
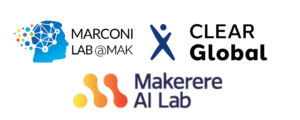
Masakhane NLU: Conversational AI and Benchmark datasets for African languages
Conversational AI and dialog systems tools have become ubiquitous. They have been very useful for many practical applications, for example: planning for travel and communicating with medical chatbots, and basic household activities like setting alarms or switching on light bulbs. However, these tools are only available for high-resource languages like English or French, because of the lack of essential datasets to power these technologies in many low-resource languages: especially African languages.
This project will develop conversational AI datasets for 16 African languages covering intent detection and slot-filling tasks needed by dialog systems to understand and reply to users’ requests. In parallel, this project will extend popular commonsense reasoning datasets like natural language inference (NLI) and the choice of plausible alternatives (COPA) from English to 16 African languages. The team hopes these benchmark datasets will encourage the development of better, multilingual, pre-trained models for African languages.
“We are very grateful to Lacuna Fund for choosing to fund our project on the creation of conversational AI and benchmark datasets for 16 African languages. We hope these datasets will encourage the development of practical voice assistant systems tailored to meet Africa’s needs and encourage the development of better, multilingual, pre-trained models for African languages.”
– David Adelani, Masakhane NLU
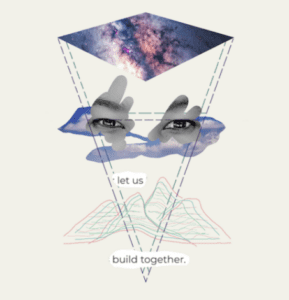
AfriHate: Hate and Offensive Speech Dataset for African Languages
Online hate is a growing problem across Africa. It inflicts harm on the people exposed to and targeted by it, pollutes and disrupts online communities and, in the worst cases, can be a precursor to physical violence. Machine learning tools that automatically find and rate the hatefulness of online content can help to address this problem, supporting content moderation efforts, social media monitoring, and threat evaluation.
However, at present there are almost no hate detection tools available for any African languages, either in academia or industry. This means that African users of online services are more likely to be subject to hate speech or unfairly have their content moderated, which can severely restrict free expression and open use of the Internet.
This project addresses this problem by introducing AfriHate, the first labeled dataset for online hate in Africa, covering 14 languages from six countries. They are also creating baseline machine learning models for each language, which will be made available to other researchers, civil society organizations, and social media platforms to use. This is a first-of-its-kind project which has the potential to transform how online hate is understood, tackled, and researched across Africa.
The AfriHate team is a collaboration between: Bahir Dar University in Bahir Dar, Ethiopia; Bayero University in Kano, Nigeria; Rewire; Masakhane; LT Group, Universität Hamburg; and, the Laboratory of Artificial Intelligence and Decision Support (LIAAD).
The team points to a statement by Nelson Mandela as inspiration for their work:
“No one is born hating another person because of the color of his skin, or his background, or his religion. People must learn to hate, and if they can learn to hate, they can be taught to love. For love comes more naturally to the human heart than its opposite.”
– Nelson Mandela, Long Walk to Freedom
If you want to find out more about AfriHate, contribute to the project, or make use of their resources, visit the project page: www.afrihate.org
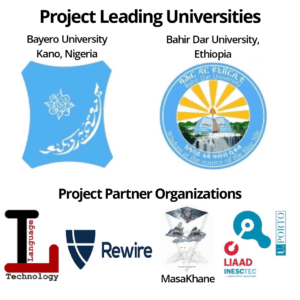
Igbo API: A Multi-Dialectal Lexical Database for Igbo
The Igbo API dataset is a robust, multi-dialectal, audio-supported Igbo-English dictionary. The team is composed of numerous lexicographers who are each experts in an Igbo dialect to ensure that the dictionary includes a wide variety of words alongside their dialectal variations.
“The Igbo API will be the largest Igbo-English, multidialectal, audio-supported Igbo-English dictionary dataset free for any type of use.”
– Ijemma Onwuzulike, Igbo API
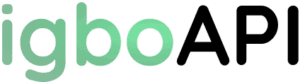
Development of Speech Corpora for Six Ethiopian Languages
This project involves the creation and augmentation of speech corpora for six Ethiopian languages: Amharic, Tigrigna, Oromo, Somali, Afar and Sidama. The datasets will be used in the research and development of an automatic speech recognition system. The team plans to develop approximately 290 hours of read speech corpora for these six Ethiopian languages.
“We work towards language-inclusive AI by developing language resources!”
– Dr. Solomon Teferra Abate, Addis Ababa University
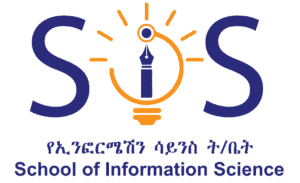
KALLAAMA
The KALLAAMA project is run by Jokalante, a Senegalese social enterprise. The project aims to produce 60 hours of audio transcriptions in Wolof, Pular, and Sérrere in order to help the community develop voice recognition solutions.
“Lacuna Fund allows Wolof, Pular, and Serer communities to access more services based on voice recognition in local languages in the near future. To ensure data quality, Jokalante will be working with Orange France, the University of Dakar, and ESP (ecole supérieur polytechnique).”
– Ndeye Amy Kebe, Jokalante
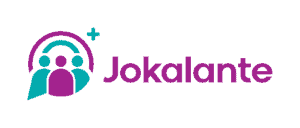
MasakhaneDAMT: African language translation dataset for domain adaptation
The quality of translation done by neural machine translation (NMT) systems depends on the availability of large amounts of in-domain parallel data used during training. However, for all language combinations, in-domain data is often sparse.
As a result of in-domain data sparsity, adapting NMT systems to new domains remains a challenge for both high-resource and low-resource languages, including many African languages. The goal of this project, therefore, is to create a large domain-specific corpus for five of the most widely-spoken African languages with at least 10,000 parallel sentences per domain. These five African languages are Swahili, Hausa, Yorùbá, isiZulu, and Amharic; they were carefully selected to include the different classes of African languages and cover all regions in the African continent.
For this project, we plan to consider two major domains, which are medical and information technology (IT) news. We chose these two domains, in particular, to provide Africans with access to public health information and IT news in their native languages.
“We are grateful that the Lacuna Fund chose to fund this project. We are excited about this project because of the potential impact it will have on the Natural Language Processing community as well as across the African continent. We anticipate the development of translation engines that can accurately translate texts from the health and IT domains from/into the selected African languages using the proposed dataset. Furthermore, this dataset will also be useful in the development of other African language technologies such as speech technologies—as most African languages are spoken languages.”
– Clement Odoje, MasakhaneDAMT
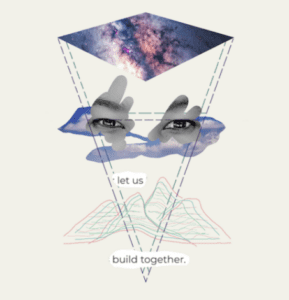